Spectra Plus Sc Keygen Generator Crack
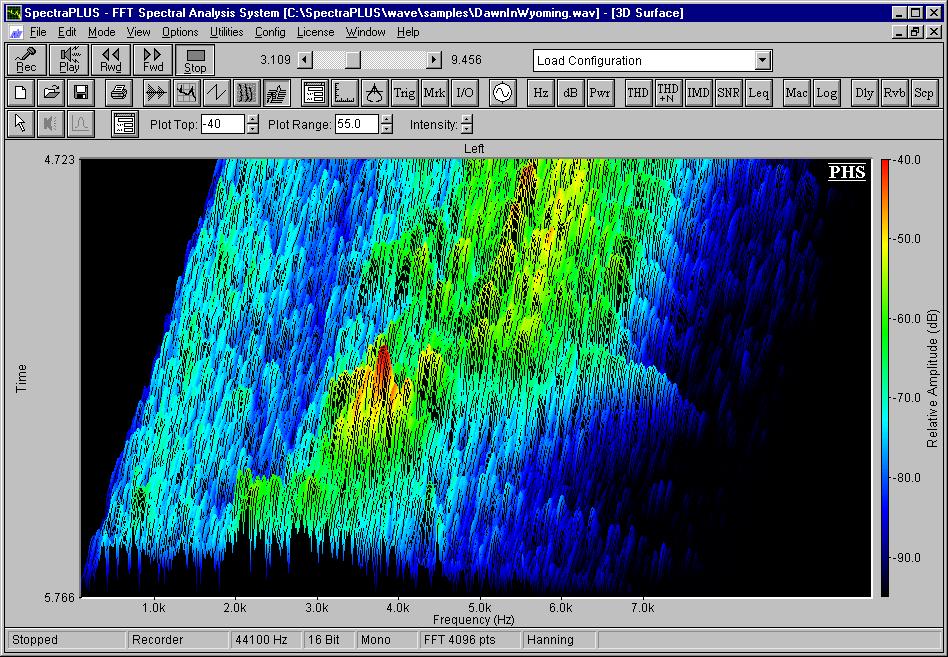
Jan 25, 2018 - Google Earth 5.0.2 serial maker ·- Deep Exploration Cad Edition 5.0 serial keygen Mapcontacts For Google Earth 2.5.0.2 serial number.
Stress corrosion cracks (SCC) in low-pressure steam turbine discs are serious hidden dangers to production safety in the power plants, and knowing the orientation and depth of the initial cracks is essential for the evaluation of the crack growth rate, propagation direction and working life of the turbine disc. In this paper, a method based on phased array ultrasonic transducer and artificial neural network (ANN), is proposed to estimate both the depth and orientation of initial cracks in the turbine discs. Echo signals from cracks with different depths and orientations were collected by a phased array ultrasonic transducer, and the feature vectors were extracted by wavelet packet, fractal technology and peak amplitude methods. The radial basis function (RBF) neural network was investigated and used in this application. The final results demonstrated that the method presented was efficient in crack estimation tasks. 1. Introduction Low-pressure steam turbine discs are critical components in power plants which rotate at high speed throughout the year.
With the increase of usage time, stress corrosion cracking may occur in the blade attachment region of the turbine discs, leading to heavy financial losses, and even severe accidents [–]. Therefore, the initial crack inspection and the forecast of their propagation are essential to the safe operation of the turbine discs, and reliable methods should be developed to serve in this task.
Ultrasonic phased array inspection technology has recently been attracting a great deal of attention in nondestructive evaluation applications []. The most desirable feature of phased array inspection is the ability of steering and shaping the sound beam flexibly, which is appropriate for detecting components with complex geometrical shapes. Yang and co-workers obtained crack depth information in the turbine discs from sector images produced by the phased array ultrasonic technique []. Paragon extfs for windows crack version.
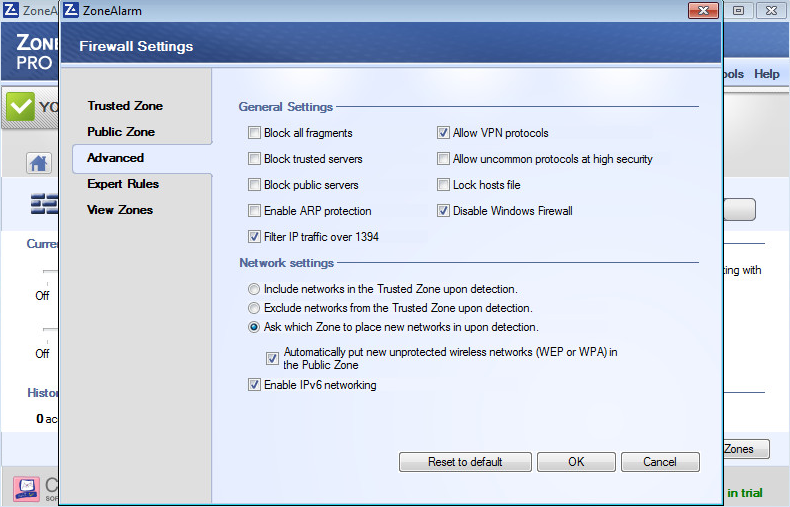
However, the orientation information of the smaller initial cracks, which is important for the estimation of the crack growth rate, propagation direction and working life of the turbine disc, cannot be distinguished effectively in the sector images. Moreover, the crack orientation can greatly influence the evaluation of crack depth, for the reason that the echo amplitudes vary from reflective surfaces with different directions. As a result, the estimations of the depth and orientation information of the initial cracks are both essential in turbine disc crack detection. There are some traditional methods for the estimation of flaw size in ultrasonic non-destructive testing. When the flaw size is smaller than the ultrasonic beam diameter, the amplitude-equivalent method is most often used, including the equivalent test specimen method and the AVG curve method; to the contrary, when the flaw size is larger than the ultrasonic beam diameter, the length testing method is always used which includes the 3, 6 and 12 dB methods. However, none of the methods mentioned above take the orientations of the flaws into account, which is an obstacle to the testing of the cracks with different orientations.
To solve this problem, crack tip diffraction signals are generally used to detect the location of the crack tip, and then the depth and orientation information can be obtained simultaneously []. However, this method has its limitations, that is, the isolation of the crack tip signal requires the face of the crack not to be oriented perpendicular to the direction of beam propagation, and commonly, the early cracks are not big enough to generate tip diffraction signals and cannot be measured in this way. In light of the above, more effective methods are needed to estimate the depths and orientations of smaller cracks in their early stage. In recent years, artificial neural networks have been proved to be effective for flaw identification and evaluation in ultrasonic non-destructive testing.